Your company’s data system is outdated, and the CEO wants to modernize—fast. The thought is daunting, like scaling a mountain with a toothpick. What if there’s a guide to turn this climb into a strategic ascent? Enter Databricks, where legacy migration leads to data leadership.
Now, let’s embark on another migration adventure! As part of a tech team, you’ve likely faced the challenge of a migration project—or perhaps several. This widespread phenomenon crosses industries, domains, and technologies, marking a pivotal step to maintaining relevance. Change is not just inevitable, but essential for business growth and to better meet customer needs. Whether implementing new ERP or CRM systems, moving from on-premise to the cloud, navigating mergers and acquisitions, transitioning from monolithic architectures to microservices-based applications, or adapting to new regulations, each scenario demands a migration effort. The common thread? A quest for superior solutions that align with the evolving demands of the business and ensure that IT strategies are in lockstep with business objectives, paving a unified path toward organizational success.
Solving modern data problems demands modern solutions. That’s where Databricks Data Intelligence Platform shines, offering unparalleled data management and data science capabilities in the AI-driven world. This blog isn’t about selling you on Databricks; we’re here to guide you through the journey to Databricks, making it as smooth and rewarding as possible, enabling efficiencies and cost savings that other platforms simply can’t match.
Data Analytics has deep roots, with the Data Warehouse becoming a staple for analytics nearly three decades ago. During this time, we’ve seen numerous technological advancements to aid decision-making through Data Warehouses, BI, predictive analytics, and ETL/ELT for data movement. These developments have shaped various strategies for data management, lifecycle governance, and security policies. Companies embarking on their journey to a new platform can originate from any point within these three decades, each adopting different methodologies.
EXPLORING DIVERSE MIGRATION PATHS
With our vast experience in data migration, we’ve seen it all. Here’s a snapshot of the diverse backgrounds companies come from on their way to Databricks:
- TRADITIONAL COMPANIES: Often on-premises or hybrid clouds, maintain their data warehouses on RDBMS systems such as Oracle, Sybase, Exadata, Netezza, SQL Server, MySQL, and Teradata. They perform ETL through once-popular commercial solutions or manage data flow with stored procedures and complex SQL queries scheduled for nightly runs. Their primary focus has been on BI reports for operational and analytical insights. Missing the upgrade wave to big data, Hadoop, and cloud technologies, these companies now find it essential to adopt advanced analytics, machine learning, and General AI to remain competitive. Their migration to Databricks, promises a future marked by advanced analytics and machine learning capabilities.
- BIG DATA PIONEERS: Companies that joined the big data wave around 2010, using vendor platforms such as Cloudera, Hortonworks, or MapR, or those who built significant on-premises clusters consisting of hundreds and thousands of nodes, are now looking to upgrade their data platforms with Databricks for better efficiency. They are well-versed in the 3Vs of Big Data (Volume, Variety, and Velocity), have integrated data into their decision-making processes, are familiar with data lakes, and likely manage both data lakes and warehouses. However, these technologies have hit a plateau in evolution alongside recent advancements in cloud technology. Now, they recognize the necessity of adopting the Lakehouse architecture, a concept pioneered by Databricks from the start.
- BORN-IN-THE-CLOUD COMPANIES: Young, “born in the cloud” companies initially thrived on cloud ecosystems for data management but later faced obstacles due to the complex architecture and the need for significant engineering effort. While scaling with cloud applications is feasible, it is not a long-term, cost-effective strategy. Moreover, certain data warehouse technologies fall behind their competitors in terms of innovation.
- CUTTING-EDGE STARTUPS: Launching their products as industry-disrupting MVPs, they soon realized the necessity of a robust data platform to become data-driven. To avoid vendor lock-in and maintain flexibility, they chose open-source tools and frameworks, along with a data lake or warehouse solution, often referred to as the modern data stack (MDS). However, as these companies expanded, they faced scalability challenges, resulting in a tangled mix of tools and custom pipelines that complicated their data infrastructure.
- TECH-FORWARD INNOVATORS: Companies that had already undergone a digital transformation and used cloud-agnostic data warehouse solutions enjoyed their modernity, speed, and innovation but were soon deterred by the high costs associated with data processing and ELT. It was like burning furniture to heat the house—the initial appeal faded as the platform became prohibitively expensive, leading to higher operational costs that could have been invested in innovation. Despite offering a comprehensive suite through partnerships and continuous feature updates, these solutions fell short in widespread adoption for AI/ML and Gen AI applications due to limited data science capabilities, primarily constrained by SQL as the native engine.
This list is not exhaustive, and some of these companies have undertaken multiple migrations in past decades to modernize their data ecosystems for better efficiency, innovation, and cost-effectiveness.
NAVIGATING THE MIGRATION JOURNEY
Transitioning to Databricks marks a pivotal moment for any organization looking to harness the power of modern data analytics. Before diving headfirst into a full-scale migration, it’s wise to navigate the waters with a Pilot or MVP phase. This initial step is not just a trial run; it’s a strategic move to ensure your organization’s unique needs align perfectly with what Databricks has to offer. At the heart of this phase lies the opportunity to put Databricks to the test, focusing on converting mission-critical workloads and exploring new use cases that were previously out of reach. Whether it’s benchmarking the platform’s scalability and performance or experimenting with streaming use cases, this phase is designed to provide a clear picture of how Databricks can transform your data strategy. We have successfully guided a diverse range of clients, from Fortune 500 giants to agile startups through their pilot of MVP phases. Time and again, we have seen organizations move from uncertainty to confidence, fully convinced of Databricks’ capabilities to meet and exceed their data analytics needs.
Lovelytics has delivered over 250+ Databricks migrations and we know success is beyond the mere transfer of data from one system to another or the restructuring of data pipelines. The strategy behind migration is a complex subject, necessitating careful consideration of numerous factors, including the specific needs of the business, optimization techniques, and the timelines for implementing new use cases. Here, we’ve only scratched the surface of the intricate decision-making process that underpins a successful transition to Databricks.
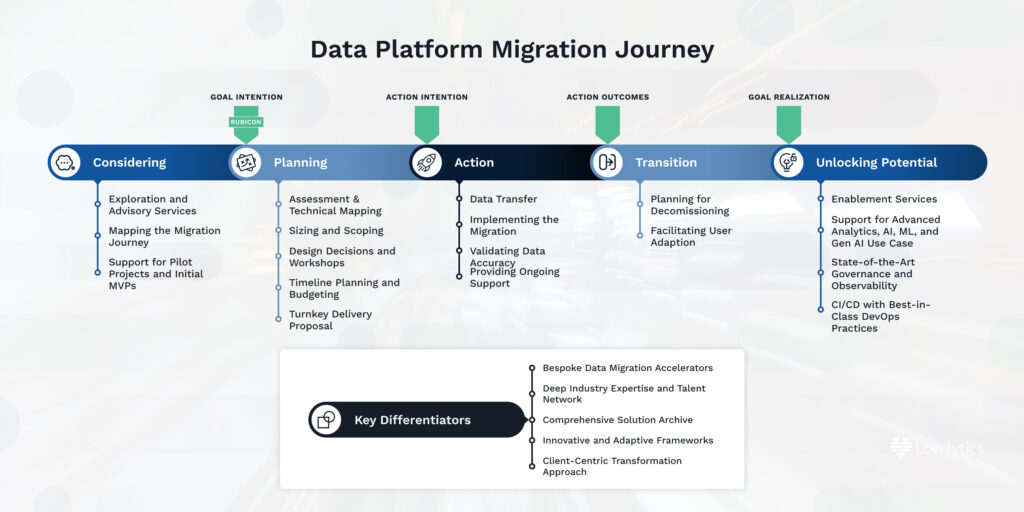
We hope the visual sneak peek into the data platform migration journey has illuminated the path ahead, with Lovelytics ready to guide you through every step. The metaphor of “crossing the Rubicon” vividly captures the essence of this transformative journey, symbolizing a decisive, exciting step to democratize data and AI by leveraging Databricks’ unified data platform with embedded AI capabilities. By migrating data to Databricks, organizations are not merely altering their data management strategies; they are embarking on a significant shift that promises to redefine how they harness their data for value. Stay tuned for our next installment in this series, where we will dive deeper into the nuances of this critical transition and how Lovelytics can ensure a smooth and successful migration to the Databricks platform.
In preparation for what lies ahead, we invite you to explore two essential reads: our blog on the Total Cost of Ownership (TCO) on the Databricks platform, and our insights into the Snowflake to Databricks migration through our Brickbuilder solution.