The View from the Lakehouse Blog Series
Contributors: Josh Adams and Andrew Sitz
Two of our previous blogs, Why We Bet on Databricks & Modernize Your Data Warehouse to a lakehouse, both focused on our technology partnerships. We discussed some technology comparisons we’ve conducted, and why Databricks’ lakehouse is now an integral part of the advisory and systems engineering services we provide to our customers. In the second of those blogs, we discussed why a lakehouse should be the foundation of a modern data and analytics architecture: “Time and money aren’t limitless. That’s why you need to feel confident that your investment choices are in alignment with your strategy.”
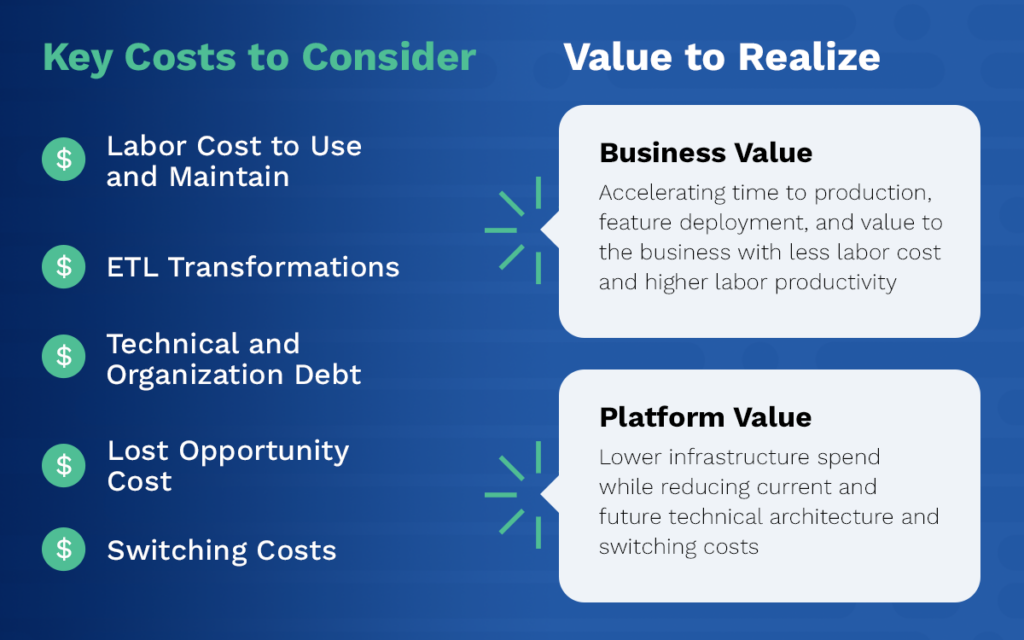
In this blog, we’ll highlight five key time and money considerations we are working for our clients that prove, time and time again, that the Databricks lakehouse is the best option to strategically align with an organization’s time and money objectives and drive maximum value over the total cost of ownership (TCO).
1. Databricks lakehouse requires the least labor cost to use and maintain
Features inherent in the Databricks lakehouse UI and pipeline development capabilities through various strategies, including Databricks’ native Delta Live Tables, notebooks, and third-party tools such as dbt reduce the amount of manual code development needed to productionize data. Our engineering teams work with our clients to rapidly deploy projects into production through Databricks’ automation features. Databricks streamlines DevOps for the data engineers and the data scientists, enabling them to do most of their own DevOps through the UI with relative ease. Databricks makes it easy to have an all-in-one or an easily-integrated solution with others capable of managing your workflows and enabling data teams to keep their focus on delivering value with data. Workflow management, scheduling, failure notifications, and data-driven alerts can all be managed natively.
More collaboration across data engineering and data science can happen in a single or minimal set of interfaces, reducing sprawl within the platform. In addition, workspaces in a Databricks environment enable collaboration across different functional user teams – data engineers and data scientists – to increase productivity on data modeling, training, and extraction. Because Databricks lakehouse combines the features needed to warehouse, model, and train data, fewer administration and maintenance hours and bodies are needed for separate infrastructure and additional platform tools.
A recent retail technology customer had a mixture of database administrators and data engineers, along with very loose definitions around these resources and a very tight budget for expanding their current workforce. As a start-up, they were at an early stage in their maturity curve and needed a sustainable solution to their previous “run fast; fix it later” framework. A mixture of SQL-only, pure python, and Spark developers were able to apply their existing knowledge directly into the Databricks lakehouse. The developers owned the entire administration process. Within two days, they were well versed in creating new workspaces and managing their compute resources. No new hires were needed. The high interoperability between open source frameworks and Databricks enabled them to adapt their existing DevOps practices directly into their Databricks environment. Their existing peer review protocol, branch forking and merging, and infrastructure – which was managed in GitLab – was integrated into their Databricks environment within one day. A complete 1:1 migration of their infrastructure, AI model, and data pipelines into Databricks was also easily accomplished within one week due to the adoption and compatibility of open source tech in Databricks. Over the next two weeks, we were able to easily implement Pandas UDFs and selective code re-writes into Spark to take advantage of distributed computing where appropriate – all without the need for additional administrators to manage the computing system.
2. Databricks lakehouse is the most cost-effective platform to perform pipeline transformations
Of all the technology costs associated with data platforms, the compute cost to perform ETL transformations remains the largest expenditure of modern data technologies. Choosing and implementing a data platform that separates consumption, storage, platform, and infrastructure – both architecturally and from a pricing perspective – enables enterprises to make more cost-effective choices for each technical layer of their solution. The Databricks lakehouse enables enterprises to optimize individual workspaces to drive down consumption costs. It also has ‘self-driving’ and self-managed/managed storage options that give enterprises a choice of where to store transformations that are driving the cost of consumption, thereby also driving down storage costs. While data storage doesn’t drive cost in the same way that ETL transformation does, an architecture built around the Databricks lakehouse enables enterprises to have complete control over and choice of data storage to make the most cost effective storage decision. There are also options for concurrent users with SQL Warehouses, rounding out the platform for downstream consumption.
We work with a variety of clients across industries that have similar needs in their modern stack. Recently, we supported a large media and entertainment customer in migrating their ETL pipelines from Snowflake to Databricks lakehouse. These pipelines encompassed data aggregation and table joins that were created from ingested JSON files, with a requirement for alerting in the event of pipeline run failures. This transition to Databricks will drive an estimated 50% cost savings for our customer.
Governing and allocating costs is also made simpler. The platform permits controllable self management that can have predictable cost forecasting and allocation. Questions like “How much does that specific process cost us?” and “Who owns that process?” are easily answered to support chargeback models from IT to business.
3. Databricks lakehouse reduces the cost and technical and organizational debt that a multi-tool architecture can incur
We have many clients who continue to leverage and administer multiple data platforms – including Snowflake and Databricks lakehouse. In these scenarios, Snowflake performs data warehousing and the Databricks lakehouse manages pipeline transformations. Our clients using the Databricks lakehouse for warehousing, pipelines, and advanced analytics have reduced platform administration overhead, seen fewer error tickets, reduced additional technologies that support point-to-point integrations, shrunk total platform consumption costs, and minimized ingress/egress fees.
Some technology companies have (or are building) what are considered added-value features and capabilities onto core warehouses because their customers don’t want to move transformations out of the platform. However, we frequently move transformations out of these warehouses for multiple customers, typically because the cost is just too high, which quickly negates the added value of these features.
Modern organizations that have data engineers and data scientists working side by side continue to drive toward architectures that have the data with, not separate from, the analytics that become the ultimate goal of the data. Table-stakes warehousing capabilities and other features in a lakehouse may surpass those same capabilities and features in a Warehouse. But only a lakehouse can future-proof the analytic and artificial intelligence needs of the business, and meet the desire for data science on all of that stored data in one platform. Collaboration (versus siloing) that is enabled through Databricks’ multi-functional, multi-purpose workspaces is what can accelerate analytics to production and increase return on investment for data platforms.
4. Databricks lakehouse reduces lost opportunity cost and time of failed projects through faster model and pipeline deployment, a shorter production lifecycle, and faster time to productivity
The emphasis on DevOps and MLOps across the platform and supporting features enables developers to prioritize productionizing data and data models rather than on code development. Developers can build pipelines in notebooks and with integrated developer environments (IDEs) to enable all team members to work with the same DevOps methods. This reduces the chances that code sitting in notebooks dies on the vine because it couldn’t reach production. Akin to feature velocity in the application development world, new features and models are brought to market at a higher velocity. The Databricks UI also increases the visibility of the project, and product owners can mitigate barriers to success through view tables and easy-to-use SQL queries. Governance support features establish domains of ownership in a centralized platform, so it’s easy to know where to go for information and understand who owns it.
Our data engineers and data scientists are excited to be working with the Databricks lakehouse, and are pushing for more training and opportunities with clients. We see this same excitement within our clients, where 20, 30, or 200 person data engineering and data scientist teams are working directly with the languages they already know and love, and with new features that reduce manual effort for low value tasks. Language interoperability across the platform can suit how most data engineering and data science teams work. For example, teams can be programmatic with Python earlier on in pipelines, then use SQL later on closer to business logic where consumption takes place.
Our clients have made investments in hiring and upskilling their workforces. Any new technology will require new platform-specific knowledge and upskilling. However, platforms built off of commonly used open-source code that can leverage multiple approaches for development help bridge this gap. We have executed dozens of engagements helping customers execute their first use cases on the Databricks lakehouse by working side by side with their teams to upskill on the platform architecture, UI, features, and tailored integration with their existing DevOps processes and technologies. Because of the lack of dependence on proprietary data and table schema knowledge, we have seen data engineers fluent in SQL or Python and data scientists fluent in Python or R at these same clients migrating and developing production ready pipelines and models within weeks – sometimes days.
5. Databricks lakehouse is the most cost-effective switching option to mitigate vendor lock-in risk and maximize investment reusability
Finally, a level of vendor lock-in exists with any technology investment, if only formally through the cost of migrating to something else. Clients who might decide in the future that the Databricks lakehouse isn’t the right solution for them will have alternatives that are more cost effective from a migration AND employee enablement perspective, due to the reusability of the open source-based languages and features across the platform. Migrating away from data platforms that leverage proprietary formats, like Snowflake, means that hundreds if not thousands of hours have been spent on code development articulating workflows, processing steps, and queries that aren’t reusable.
“Databricks believes in openness in virtually all portions of its offerings. Delta Lake is the open-source lakehouse storage format that can be transparently accessed regardless of the compute platform. Unity Catalog enforces data governance for any client or through Delta Sharing, which is the open protocol to exchange large datasets between various platforms. Databricks’ open philosophy delivers benefits now and into the future as a protection against proprietary lock-in.” (2022 Gartner Magic Quadrant for Cloud Database Management Systems)
Databricks lakehouse provides the best value and lowest TCO
We have customers that experience cognitive bias during the technology selection process. They make choices based on what looks and feels like what they are used to, which can inhibit their advancement beyond current problem sets, and make it difficult to focus on what matters most: empowering users to make well-informed decisions fueled by data well into the future. We often see customers with a data warehousing background choose a modern data platform because it has the look and feel of a traditional data warehouse.
While the Databricks lakehouse offers the most cost-effective option for the things that drive hard dollars on an invoice – consumption, storage, platform, and infrastructure – TCO should also consider labor costs, opportunity costs, architectural costs, and switching costs. And across all of these cost considerations, the Databricks lakehouse continues to be the most strategic investment and value enabler.