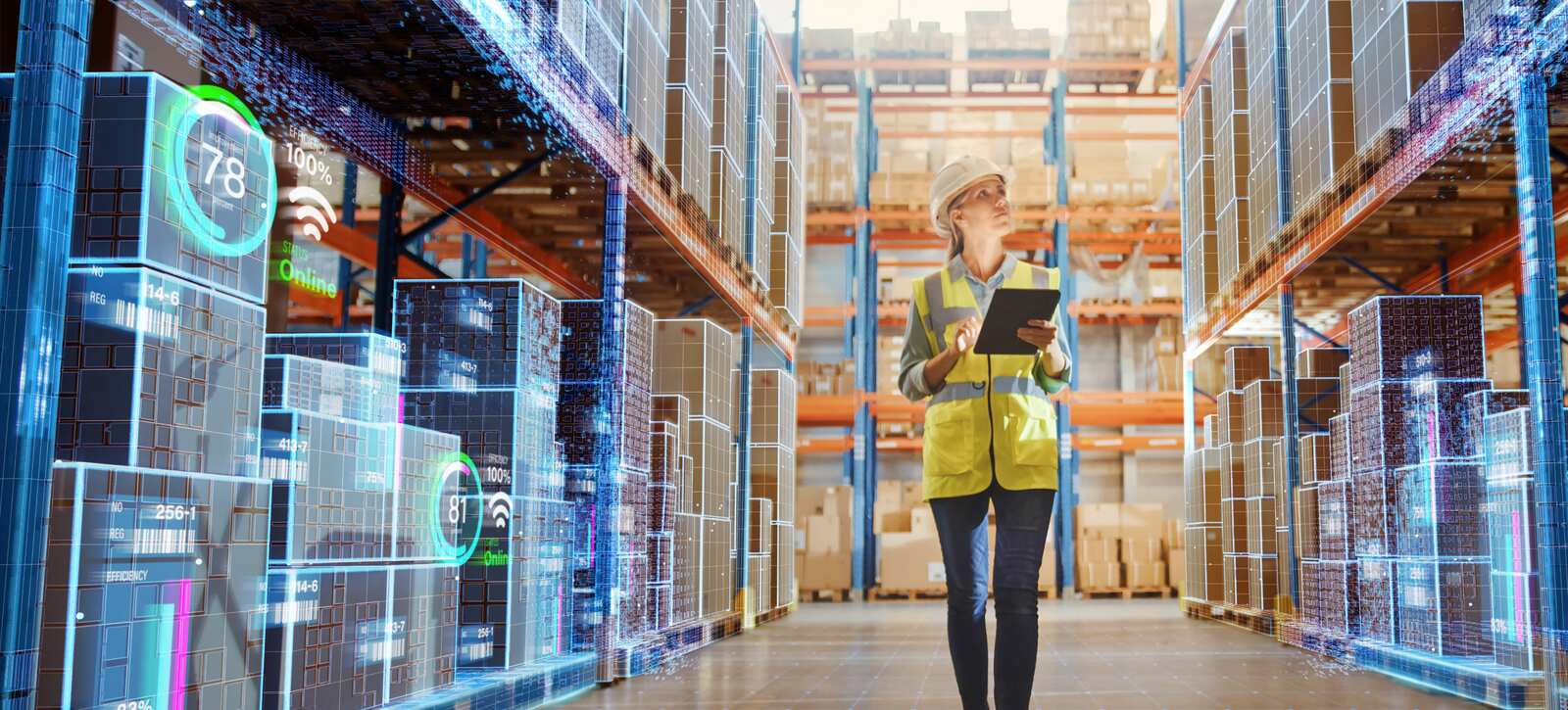
The Common Ground Between Manufacturing, Retail, and Consumer Packaged Goods Is Driving Advanced Analytics and GenAI Innovation and Investment
Author: Bill Martin
As I approach the 30th year of my career, my experience in manufacturing, distribution, logistics, and professional services working with clients in retail and consumer packaged goods (CPG) has given me a deep understanding of each. Over the past few months, I have been formulating a theory that some may see as controversial, but I see as obvious and extremely hard to refute. These businesses seem very different at first glance, but despite their outward differences, the manufacturing, retail, and CPG industries share striking similarities. These sectors are all deeply involved in product design and sourcing, supply chain management, and omni-channel sales. Each has a simple goal of manufacturing or buying a product, inventorying that product, marketing that product, selling that product, shipping that product to the customer, and getting paid for that product.
This common business model could and should be the basis for volumes of research and analysis on how to leverage best practices and create efficiencies for business processes and operations. Combining lessons learned in each industry can help develop a universally accepted and applicable operating philosophy. However, since I am a technical consultant, I will be looking at how these commonalities can drive a deeper understanding of how to leverage modern data analytics, GenAI, and machine learning to accelerate the ability of technical solutions to drive deep impact and value across all these industries, thereby creating high levels of maturity much quicker than if these problems were solved in silos. This blog post explores these commonalities, examines why these industries are traditionally viewed as separate, and highlights how modern technologies can drive cross-industry innovation and efficiencies.
Common Data, Analytics, GenAI, and Machine Learning Use Cases
The convergence of manufacturing, retail, and consumer packaged goods industries opens up a wealth of opportunities for leveraging advanced data analytics, GenAI, and machine learning. These technologies address shared challenges and drive innovation across sectors. The following use cases illustrate how these cutting-edge technologies can optimize various aspects of product design, supply chain management, sales, quality control, and customer personalization, leading to significant benefits and efficiencies.
1. Product Design and Sourcing:
Use Case: All three industries focus on designing and sourcing products, whether raw materials or finished goods. There are many examples of organizations that manage the end-to-end supply chain from raw materials sourcing through to end-consumer sales.
Technology:
• GenAI: Assists in creating design prototypes and optimizing sourcing strategies by analyzing market trends and consumer preferences.
• Machine Learning Models: Forecast demand, identify the best suppliers, and optimize the sourcing process by analyzing historical data and market conditions.
Benefits:
• Enhanced Innovation: Accelerates the design process and fosters innovation by providing data-driven insights.
• Cost Efficiency: Optimizes sourcing strategies to reduce costs and improve supplier relationships.
• Market Responsiveness: Enables rapid adaptation to changing market trends and consumer demands.
2. Supply Chain Management:
Use Case: Efficient supply chain management is a core competency across these industries. Effective management from source to consumer has a direct effect on overall business performance. Timely delivery of raw materials and finished goods must be optimized across their networks.
Technology:
• Predictive Analytics: Uses historical data and trends to forecast demand and optimize inventory levels.
• Real-Time Data Monitoring: IoT sensors and real-time data analytics track the movement of goods, monitor conditions, and provide real-time visibility into the supply chain.
• Machine Learning Algorithms: Optimize routes, reduce costs, and improve logistics efficiency by analyzing transportation data and identifying bottlenecks.
Benefits:
• Operational Efficiency: Reduces waste, lowers inventory costs, and ensures timely delivery.
• Improved Decision Making: Provides actionable insights for better supply chain planning and management.
• Customer Satisfaction: Enhances service levels and meets customer expectations through reliable delivery schedules.
3. Omni-Channel Sales:
Use Case: Selling products through multiple channels, whether to commercial clients or individual consumers, is a shared focus.
Technology:
• Advanced Analytics: Analyzes customer behavior across channels to understand preferences and buying patterns.
• Machine Learning Models: Personalize marketing efforts and optimize the overall shopping experience by predicting customer needs and tailoring recommendations.
• GenAI: Generates personalized content and recommendations to enhance customer engagement.
Benefits:
• Customer Engagement: Improves customer satisfaction and loyalty by providing a seamless and personalized shopping experience.
• Increased Sales: Boosts sales through targeted marketing and personalized offers.
• Market Penetration: Expands reach by effectively managing and integrating multiple sales channels.
4. Quality Control and Predictive Maintenance:
Use Case: Ensuring product quality and minimizing downtime is essential for all three industries.
Technology:
• IoT Sensors: Collect data on equipment and product performance, providing real-time monitoring and alerts.
• Machine Learning Models: Predict maintenance needs and optimize production processes by analyzing performance data and identifying patterns indicative of potential failures.
Benefits:
• Reduced Downtime: Prevents unexpected equipment failures and production stoppages.
• Quality Assurance: Ensures high-quality outputs by maintaining optimal operating conditions and promptly addressing issues.
• Cost Savings: Lowers maintenance costs and extends the lifespan of equipment through proactive maintenance.
5. Customer Insights and Personalization:
Use Case: Understanding customer behavior to tailor offerings and improve satisfaction is a common goal.
Technology:
• Machine Learning Algorithms: Analyze transactional data, social media interactions, and browsing behavior to uncover insights into customer preferences and trends.
• GenAI: Creates personalized marketing campaigns and product recommendations based on these insights, enhancing customer engagement and loyalty.
Benefits:
• Enhanced Customer Experience: Delivers personalized experiences that meet individual customer needs and preferences.
• Increased Retention: Boosts customer loyalty and retention through targeted marketing efforts.
• Market Intelligence: Provides deep insights into customer behavior and market trends, informing product development and marketing strategies.
Reasons for Industry Segregation
Despite their commonalities, manufacturing, retail, and CPG are traditionally viewed as distinct sectors. This separation is driven by various factors including unique regulatory environments, economic reporting requirements, international trade regulations, and the commercial strategies of technology providers. Understanding these factors helps explain the industry’s traditional segmentation and the unique challenges each sector faces.
1. Government Regulations:
• Unique Regulatory Environments: Each industry faces specific regulatory challenges. Manufacturing deals with stringent safety and environmental standards, retail with consumer protection laws, and CPG with food and drug safety regulations.
• Compliance Requirements: These regulations necessitate tailored compliance strategies, contributing to the perceived separation of these industries.
2. Economic Reporting Requirements:
• Distinct Reporting Standards: Economic reporting requirements differ across industries, influencing how financial and operational data are tracked and reported.
• Industry-Specific Metrics: Each sector uses specific metrics and key performance indicators (KPIs) relevant to their operations, reinforcing their segregation.
3. International Trade Regulations and Standards:
• Global Operations: International trade regulations and standards vary widely, with manufacturing focusing on export controls, retail on cross-border e-commerce rules, and CPG on international labeling and packaging standards.
• Adherence to Standards: These differing standards require industry-specific strategies to manage compliance, further segmenting these sectors.
4. Commercial Segregation by Technology Providers:
• Targeted Solutions: Technology providers often package similar products and services differently for each industry to create more targeted solutions.
• Increased Sales Opportunities: This intentional segregation generates additional sales opportunities by addressing the unique needs of each sector.
Leveraging Shared Technologies for Cross-Industry Benefits
Understanding the commonalities can help these industries leverage shared technologies for greater efficiencies:
1. Unified Data Platforms:
• Data Sharing and Collaboration: Implementing unified data platforms facilitates data sharing and collaboration across departments and industries, breaking down silos and enabling comprehensive data analysis.
• Integrated Insights: These platforms provide integrated insights that drive strategic decision-making and operational improvements.
2. Cross-Industry Best Practices:
• Innovation and Improvement: Adopting best practices from each other can drive innovation. For example, retail customer analytics can enhance manufacturing’s understanding of end-user needs, while manufacturing’s predictive maintenance can improve retail logistics.
• Efficiency Gains: Sharing best practices lead to efficiency gains and improved performance across all sectors.
3. Collaborative AI Initiatives:
• Accelerated Technological Advancements: Joint AI initiatives can accelerate technological advancements. Developing GenAI models for supply chain optimization or customer personalization can benefit all three industries, reducing costs and enhancing performance.
• Shared Resources: Collaborative efforts maximize resource utilization and drive collective progress.
Harnessing Shared Strengths: Driving Value Through Cross-Industry Collaboration in Manufacturing, Retail, and CPG
The manufacturing, retail, and consumer packaged goods industries, while distinct, share significant commonalities that can be leveraged to drive value across each sector. By recognizing these similarities and adopting shared data, analytics, GenAI, and machine learning technologies, these industries can optimize their operations, improve customer experiences, and achieve greater efficiencies. Understanding the reasons for their traditional segregation, whether due to regulatory requirements, economic reporting, or commercial strategies by technology providers, can help us appreciate the unique challenges each faces while also highlighting the vast potential for cross-industry collaboration and learning.
Ready to revolutionize your operations? Contact us today to explore how our cutting-edge solutions can elevate your business to new heights.